Addressing the new wave of the IoT with Edge AI
By Omar Cruz, Senior Manager, Product Management PSoC™ Edge, Infineon Technologies
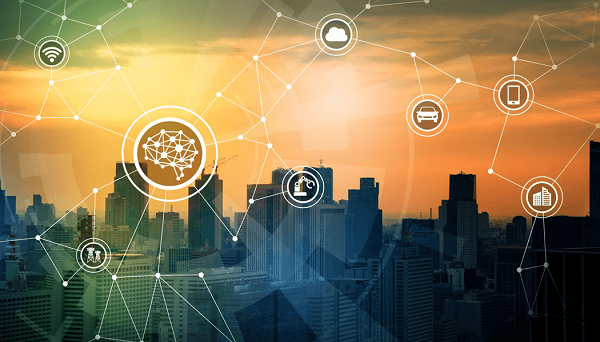
Currently, we are observing the rapid expansion of the Internet of Things (IoT). Every second, 127 devices are being connected, and it is projected that by 2027, there will be a staggering 43 billion IoT devices*. As this market continues to grow and develop, there is an increasing demand for advanced, efficient, and precise system solutions that can enhance our daily lives. Among the numerous pivotal technologies that contribute to this promising future of the IoT, Edge AI plays a vital role in augmenting the capabilities of IoT. By enabling data analysis, predictive insights, and intelligent decision-making at the edge of the IoT, Edge AI empowers the IoT ecosystem.
Let’s first begin with the basics – what is Edge AI?
Developers and users may have a good understanding of Artificial Intelligence (AI) and/or Machine Learning (ML), but the concept of Edge AI is not as widely recognized. Edge artificial intelligence (Edge AI) involves the utilization of AI applications in an edge computing setting or device situated near the data source, as opposed to a centralized environment like a cloud computing facility.
In practical terms, Edge AI encompasses the gathering of data from sensors or alternative sources like trackers and health monitoring devices. This data is then processed locally on the edge device using an AI model. The resulting output from the model is utilized to initiate an action or send a notification. By processing data locally, Edge AI enables quicker inference and facilitates real-time applications. It also reduces latency and network congestion, enhances privacy and security, and improves energy efficiency.
Nowadays, developers are exploring various use cases and applications for Edge AI, including facial/gesture recognition in appliances and smart home systems, wearables, and health monitoring devices. Additionally, they are implementing predictive maintenance in factory automation and utilizing AI to identify and address suspicious activity in real-time, ultimately leading to more cost-effective and efficient services.
AI is commonly deployed in various applications such as smart speakers and voice assistants, where speech recognition analysis plays a crucial role. This involves utilizing a combination of advanced AI technologies, including automatic speech recognition (ASR) to translate sound waves into words, Natural Language Understanding (NLU) to derive meanings from those words, and Natural Language Generation (NLG) for the smart speaker’s responses.
The smart home market is witnessing additional trends that are set to revolutionize the efficiency of edge devices and enhance user experiences. For instance, a possible use case may include washing machines that can adjust the water and detergent amount, as well as the rinsing and spinning times, to allow more efficient washing. Thermostats can learn users’ preferred temperatures, detect indoor and outdoor temperatures, and even recognize the individuals present in the room. Ovens may become capable of personalizing meals based on users’ taste preferences, while also providing safety measures by restricting usage to adults only. Furthermore, vacuum cleaners can differentiate between different floor types and optimize cleaning techniques and battery efficiency accordingly. All of these use cases rely on sophisticated AI algorithms implemented at the edge.
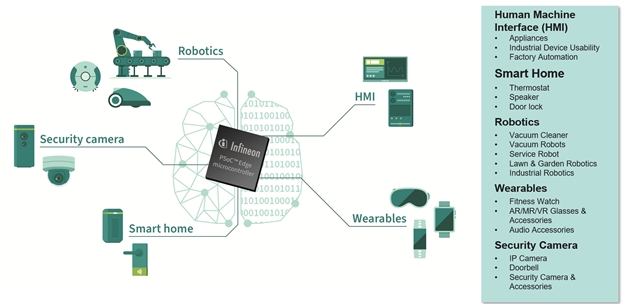
It is customary for a novel trend or technology to introduce fresh obstacles and crucial elements to consider, and Edge AI is no different in this regard. What is evident at present is that Edge AI significantly influences the subsequent domains.
– Higher Performance (and Low Power)
The expansion of the Internet of Things (IoT) leads to an increased demand for additional sensors, which consequently leads to a greater sharing of information. This, in turn, enhances the complexity of devices, necessitating a greater need for enhanced computing capabilities. Now, with the added capability of processing machine learning (ML) operations directly on the device itself, the requirement for a high-performing core and a neural net compute hardware accelerator becomes indispensable for this new generation of edge devices. This demand becomes even more challenging when we consider the need for power optimization, allowing that battery-operated and power-conscious end devices can operate efficiently while consuming minimal power. The advantage of this approach is that it consumes less energy by storing data and running algorithms on the edge device, as opposed to transferring everything to the cloud.
– Security and Privacy
Despite the fact that Edge AI devices carry out most of their operations and data processing locally, reducing security threats and privacy concerns by minimizing data transmission to the cloud and external locations, it should be noted that not all data stored in these devices is automatically safeguarded against attacks. As security threats advance, it becomes imperative to implement a strong and appropriately tailored level of embedded-security across Edge AI devices to ensure the preservation of data integrity and privacy.
– Lack of expertise, time consumption, and comprehensive enablement
Developing Edge AI devices without the necessary expertise is an extremely challenging task. This difficulty can arise from a lack of understanding about how to utilize specific accelerators and processors that are optimized for AI/ML, as well as a lack of experience in utilizing software for the development and deployment of AI models. Insufficient knowledge and the time-consuming nature of the process can hinder developers from choosing the most effective approach and restrict management from making informed decisions.
Undoubtedly, when faced with such scenarios, the presence of a comprehensive, fully-integrated hardware and software package along with an end-to-end toolchain solution offered by professionals can greatly minimize ambiguity and accelerate the time to market for the upcoming generation of Edge AI devices.
Infineon’s PSoCTM Edge tackles the challenges of Edge AI by unveiling a fresh line of microcontrollers equipped with a wide range of features such as top-notch performance, low power consumption, cutting-edge security, and extensive support to expedite the time to market.
As mentioned, the growing complexity of systems, including the aggregation of sensors and the handling of complex data at the edge, is challenging the performance capabilities of microcontrollers. Simultaneously, there is an ongoing demand for low-power consumption and high energy efficiency in the IoT industry. To support these requirements, Infineon has introduced a multi-domain architecture approach with PSoCTM Edge offering high performance capabilities, including a high-performance core and a hardware accelerated neural processor unit, while also supporting increased energy efficiency by featuring an ultra-low-power domain for Always-On applications. This allows an IoT device to remain in deep sleep mode while being able to detect acoustic events or face detection actions and trigger actions so the system can fully wake-up, perform the task required and go back to sleep, maximizing energy efficiency resulting in longer battery life without sacrificing performance. Thus, Edge AI does not only accelerate digitalization but also helps support decarbonization via power optimization.
As previously stated, an additional significant obstacle is providing appropriate data protection and reducing security risks. Therefore, it is imperative for solution providers to enhance the security measures of their Edge AI solutions, enabling the availability of more secured devices for consumers. Without adequate protection, Edge AI devices can be manipulated, potentially serving as an entry point into the network. Moreover, attackers can exploit the widespread availability of Edge AI enabled devices such as thermostats, smart speakers, or smart locks, by analyzing them for vulnerabilities and developing malicious software to compromise both the technology and the network. For these reasons, having a robust, right-sized embedded security architecture – like PSoCTM Edge has introduced – becomes paramount for these new wave of Edge AI devices.
Lastly, Infineon understands the importance of time to market for an Edge AI device. With its recent acquisition of Imagimob, Infineon also now adds the capability to offer an end-to-end ML platform with high flexibility and ease of use, with a strong focus on delivering production-grade ML models. With strong ecosystem partners, comprehensive documentation, evaluation kits with connectivity and HMI modules, as well as the industry-recognized ModusToolbox™ software, integrated with Imagimob’s Edge AI development platform and its Ready Models for a faster and validated way to take ML-enabled microcontrollers to production, PSoC™ Edge provides the right hardware, software and tools offerings for a friction-free design experience and an accelerated time to market.
Learn more about how Infineon makes the next generation of Edge AI devices possible.