Courtesy: NVIDIA
Creating New Drugs, Faster
Alzheimer’s. Cancer. Parkinson’s. These devastating diseases are just a few of the hundreds that scientists are struggling to cure with new medicines in the face of soaring drug-discovery costs and testing times.
Now, artificial intelligence is showing the potential to be a faster, more efficient way to find and develop new drugs. A growing number of companies and university researchers are tackling some of medicine’s toughest problems by using AI computing to predict which drug molecules are most likely to be effective treatments.
“This is a revolution in the pharmaceutical industry,” said Alex Zhavoronkov, CEO of Insilico Medicine, which uses GPU-accelerated deep learning to target cancer and age-related illnesses.
Drug Discovery Now: It’s Broken
The revolution can’t come soon enough. Developing a new drug costs an average of nearly $2.6 billion and can take as long as 14 years, according to figures from a Tufts University study and the U.S. Food and Drug Administration.
Even then, less than 10 percent of potential medicines make it to market, said Jackie Hunter, CEO of BenevolentBio, the life sciences arm of London’s BenevolentAI.
“The economics are not sustainable,” she said “We need to think about doing things differently.”
AI Drug Discovery
BenevolentBio aims to reinvent drug discovery by using deep learning and natural language processing to understand and analyze vast quantities of bioscience information — patents, genomic data and the more than 10,000 publications uploaded daily across all biomedical journals and databases.
“Humans alone cannot process all this information to advance scientific research,” Hunter said.
BenevolentBio’s deep learning software, powered by the NVIDIA DGX-1 AI supercomputer, ingests and analyzes the information to find connections and propose drug candidates.
Hunter’s team also uses deep learning to generate molecules with properties that are likely to be effective against specific diseases, such as new molecules for neurodegenerative diseases, but without adverse cardiac or liver side effects.
So far, BenvolentBio has identified two drugs for amyotrophic lateral sclerosis (better known as ALS) — a progressive neuro-degenerative disease — that in its tests worked better than those on the market, Hunter said. After it obtains patents, she said BenevolentBio will approach pharmaceutical companies to pursue development.
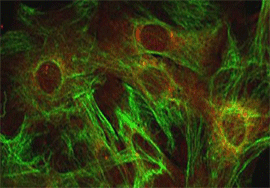
Imagining a Molecule
Today, pharmaceutical companies must screen large numbers of molecules for drug candidates, then painstakingly test each in the hope of finding a winner. This expensive process takes enormous amounts of time.
Baltimore’s Insilico Medicine is working to flip this dynamic on its head by “imagining” new cancer-like molecules with specified properties. They do this with a relatively new deep learning technique known as a generative adversarial network (GAN).
GANs can create entirely new data that is indistinguishable from real data by using two competing neural network models. A generative model tries to generate output that “looks like” real data. A discriminative model takes input from both the generative model and real data and tries to distinguish between them.
Generative models are commonly used to create images, speech or text, but this is the first time a GAN has been used for cancer drug discovery, Insilico said.
Molecules on Demand
In their recent paper, researchers in Insilico’s Pharma AI division describe how their network used historical biological and chemical data to imagine 69 new molecules with the potential to fight cancer.
“Rather than using trial and error when looking for molecular leads, you can tell the network to generate those leads and it will do it on demand,” said Insilico’s Zhavoronkov. Insilico is now extending this work to imagine antimicrobial leads.
Insilico trained its algorithms using the CUDA parallel computing platform, Tesla K80 accelerators and TITAN X GPUs with cuDNN on the Theano and TensorFlow deep learning frameworks.
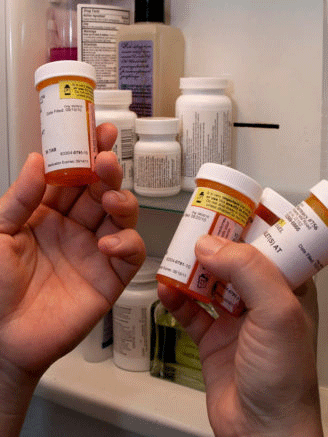
Molecules by Design
Alán Aspuru-Guzik, a Harvard University professor of chemistry and chemical biology, is also seeking to build treatments from the ground up using a deep learning neural network for what he calls “inverse molecular design.”
To find candidates for drug development, the deep learning software proposes novel molecules that posses certain properties, Aspuru-Guzik said. The software also generates new molecular structures by combining properties of existing drugs.
These methods might accelerate drug discovery, Aspuru-Guzik said. He describes his research using a generative model for automatic chemical design in a recent paper published in ArXiv, the online archive of research articles.
Aspuru-Guzik trained his deep learning software on 250,000 drug-like molecules using the CUDA parallel computing platform, NVIDIA Tesla K80 GPU accelerators and cuDNN with the Theano deep learning framework.
No one expects AI drug discovery to replace scientists. Most believe it’ll supplement their skills, and that all discoveries must be validated by people.
“This is an exciting time to be at the intersection of artificial intelligence and the pharmaceutical industry,” said BenevolentBio’s Hunter. “I hope to use this to get more medicines to patients faster.”