AI simulations automatically design geometries to minimize energy loss
TOKYO, May 30, 2018 – (JCN Newswire) – Fujitsu Laboratories Ltd. and Fujitsu Limited today announced the development of technology that can automate the design of magnetic material geometries, using AI to minimize energy loss. To design magnetic materials, which are common components in electronic devices, developers previously worked under conditions where they had to spend large amounts of time and money conducting tests based on trial and error. The new technology automates and digitizes the development of prototypes, significantly improving R&D efficiency. In addition, this technology is expected to enable geometric designs for magnets, even without specialized expertise, in a variety of R&D fields, such as power electronics and electric vehicle motors. Fujitsu aims to make design services incorporating this technology available through the cloud in 2020.
Development Background
Magnetic materials, which become magnets when a magnetic field is applied, are used in a variety of components and devices, including electric vehicle motors and inductors, which store electrical energy in the power sources of electronic devices. However, there will always be some magnetic loss, where a portion of energy is lost as heat, due to magnetism. This magnetic loss can change significantly based on the geometry of the magnetic materials, and is directly linked with the energy efficiency of the component or device. Therefore, in order to deliver high energy efficiency, it has become important to design the geometries of magnetic materials with consideration for magnetic loss.
Issues
With existing design methods for the geometries of magnetic materials, because of the strong non-linearity unique to magnetic materials (magnetic hysteresis), it is difficult to find an optimal geometry for minimizing magnetic loss, thereby making automated geometry design challenging. In addition, even when a completed design is simulated, there can be errors in the magnetic loss simulation that differ by several multiples or even several orders of magnitude from experimentally measured values, inhibiting the sufficient and accurate predictions for design development. As a result, magnetic material geometry designs had to rely on the experience and insight of designers as well as experimental trial and error, requiring substantial expenses of time and money.
About the Newly Developed Technology
Through the use of AI, Fujitsu and Fujitsu Laboratories have now developed technology to automate the design of geometries that minimize magnetic loss in virtual space. Details of the newly developed technology are as follows.
1. Technology to Accurately Simulate Magnetic Loss
With this technology, it has become possible to accurately compute the distribution of eddy currents(1) that flow through an inductor by formularizing the dielectric effects of ferrite microstructures, which are the main magnetic materials used as inductor materials. This makes it possible to accurately compute the size of eddy current loss, which is the primary cause of ferrite magnetic loss. Accordingly, while it was difficult for previous estimation methods to estimate the size of the eddy current loss when the inductor’s operating frequency exceeded a few dozen kilohertz, the new technology has enabled the estimation error for magnetic to be below 10% across a broad range of operating frequencies, up to several megahertz (figure 1).
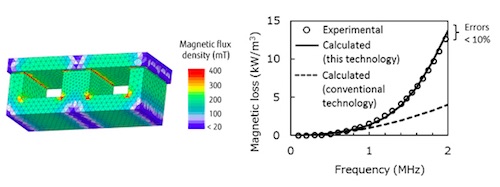
http://www.acnnewswire.com/top
Figure 1: (Left) Simulation of the magnetic loss of an inductor (distribution of the density of magnetic flux within the magnetic material); (Right) Comparison of experimental and simulated results
2. Technology to Optimize Design Using AI
By linking the newly developed magnetic loss simulation with a genetic algorithm(2), Fujitsu Laboratories and Fujitsu developed a formula to automatically find geometric parameters (dimensions for each part of the magnetic material’s shape) that minimize magnetic loss. The companies have now developed a technology to calculate the collection of optimal solutions (a Pareto-optimal front, 3) that with speed and stability minimizes magnetic loss by exercising control in consideration of magnetic characteristics, so as to maintain a proportion of superior individual items above a certain threshold of excellence, for respective generational change that brings about a next generation. For example, it is desirable for both the physical size and the magnetic loss of an inductor to be as small as possible. However, when inductance, which is the primary electrical characteristic of an inductor, is restricted to a certain level, there is a trade-off between the two. In that case, this technology is capable of automatically finding the set of optimal designs (figure 2).
http://www.acnnewswire.com/top
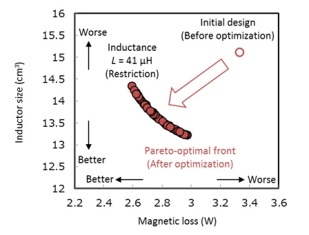
Figure 2: Results of automated design of an inductor (each dot corresponds to a single inductor geometry)
Effects
Using the newly developed technology, Fujitsu Laboratories and Fujitsu were able to automate the experimental design of magnetic material geometries in a virtual space. As a result of internal trials and when designing the shape of an inductor using ferrite materials, this technology made it possible to complete the development of prototypes in a few days, which would have taken several months beginning with the creation of multiple types of molds. In this way significant streamlining of R&D was made possible.
Future Plans
Fujitsu Laboratories and Fujitsu aim to make design services incorporating this technology available through the HPC cloud for scientific calculations in fiscal 2020. In addition, they aim to further develop this AI technology, enabling the creation of magnetic design geometries without specialized expertise by linking it with materials informatics(4).
(1) Eddy currents When magnetic flux changes in a conductor of electricity, it can induce a vortex-shaped current within the conductor, according to the laws of electromagnetic induction. The heat generated by the flow of eddy currents turns into energy loss. The location and size of eddy currents occurring within magnetic materials are determined by the material’s geometry and its electrical properties.
(2) Genetic algorithm A computational method of optimization modeled on the workings of biological evolution. For a current generation of possible solutions, multiple copies are made, which are then crossed with one another and mutated, and then “survivors” are selected to create the next generation of solutions. By repeating this process, the method finds better solutions. The desired evolution is encouraged on the basis of selecting “survivors” on the degree to which they suit the appropriate parameters.
(3) Pareto-optimal front When one wants to minimize multiple values that have a trade-off relationship, and when no circumstances exist that would provide smaller values for all variables than a certain set of parameters, those parameters are called the Pareto optimum. Typically multiple Pareto optimums exist, and the line or plane formed by those optimums is called the Pareto-optimal front.
(4) Materials infomatics An effort to accelerate the search for new materials by combining and fusing technologies in the materials field, such as materials synthesis and analysis technologies and materials simulation, with such technologies as data science and AI. It is expected to significantly reduce the time and cost required for materials development.