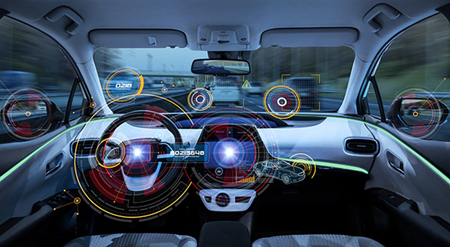
By Carrie Gan, Mouser Electronics
The dawn of autonomous vehicles is upon us, revolutionizing how we use and perceive mobility. Presently, we are witnessing a paradigm shift in the automotive sector, wherein vehicles are evolving from standalone mechanical entities operated by humans to interconnected intelligent systems capable of autonomous travel. This shift promises to transform our streets into a network of self-driving vehicles, reducing human error and potentially enhancing road safety.
However, it is important to acknowledge that undertaking this journey is not without challenges, as it demands notable advancements in technology, infrastructure, and legislative frameworks. With the exciting new era just around the corner, we must fully comprehend the profound implications of this transformative shift and undertake the necessary measures to adapt to a future where our vehicles assume control, thereby reversing the traditional dynamic between the driver and the vehicle.
Exploring the Current Landscape of Self-Driving Vehicles
The U.S. Society of Automotive Engineers, Inc. (SAE) established six levels of vehicle autonomy in 2016. These levels, ranging from 0 to 5, determine the degree of automation in vehicles and serve as crucial milestones in the evolution of fully automated driving. Each level represents a different level of human involvement and system autonomy, providing a framework for understanding the capabilities and limitations of automated driving systems.
Currently, the majority of vehicles on the road are operating at level 0 or 1, indicating a lack of autonomous operation or merely minimal driver assistance features, such as cruise control. Particularly within the Electric Vehicle (EV) market, there has been a significant increase in the number of vehicles operating at level 2, which is characterized by partial automation. In these vehicles, the system assumes responsibility for managing sub-tasks concerning both forward and lateral motion, although human supervision remains critical.
2024 marks a significant milestone in the journey towards full autonomy, with level 3 autonomous vehicles starting to hit the roads. Mercedes-Benz, for instance, has made significant strides in this area. Their 2024 EQS Sedan and S-Class models are the first to feature L3 autonomous driving systems in the United States. These advanced systems empower vehicles to handle the majority of driving tasks autonomously, requiring driver intervention only in particular scenarios, such as challenging road conditions or instances where the autonomous system fails to perceive its surroundings accurately.
In 2019, an estimated 31 million cars worldwide were equipped with varying levels of automation. By the end of 2024, the market is projected to see over 54 million vehicles featuring some level of automation, surpassing the current market share . Furthermore, research conducted by IHS Markit suggests that by 2025, the market share of level 2 and 3 mass-produced models will surpass 50%, with over 8 million vehicles shipping with level 3, 4, or 5 autonomous operations .
As consumer interest continues to grow, the demand for robust legislation also plays a crucial role in shaping the landscape of autonomous vehicles. In the United Kingdom, recently revised Automated Vehicles Bill in February 2024, outlines the government’s strategy to evaluate automotive regulations and establish a legal framework for safely introducing self-driving vehicles in Great Britain . The European Union is currently in the process of approving the sale of fully self-driving cars, which is part of a larger initiative to enhance car safety standards. This move is expected to position the EU as the first global market to authorize the sale of Level 4 autonomous vehicles legally .
As we look towards the future, it is evident that the journey towards increased autonomy has begun with the introduction of supportive laws and a rapidly growing market. Nonetheless, unresolved technical challenges persist and require diligent attention.
Research institute IHS Markit predicts that by 2025, the market share of L2+L3 mass-produced models will exceed 50%. Now, every automotive company is working at full capacity on research and development to promote the mass production of higher-level autonomous driving models.
Higher Autonomy Requires Increased Computing Power
The consensus among major car manufacturers and autonomous driving chip manufacturers is that high computing power is crucial in the competition for autonomous driving.
Consider Tesla as an example; besides manufacturing cars, the company has started allocating substantial resources to AI training and developing its proprietary supercomputers. July 2023 marked a significant milestone for Tesla as they began mass-producing their indigenous supercomputer Dojo. Tesla envisions that Dojo will rank among the world’s five most advanced supercomputers by 2024, with a total computing power of 100 exaFLOPS targeted to be achieved by October 2024 .
Why Do Car Manufacturers Need Supercomputers?
When Tesla first announced the Dojo project in 2021, Elon Musk emphasized that “the only way to solve self-driving is to solve real-world AI”. According to Musk and many other industry experts, solving the problem of autonomous driving will be challenging for companies unless they possess robust AI capabilities and powerful supercomputers.
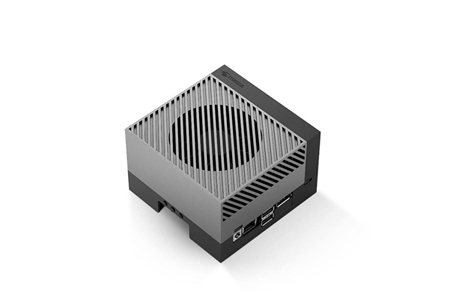
An analysis of the current market landscape of autonomous driving chips reveals that Mobileye, backed by Intel, holds a dominant position in the Advanced Driver-Assistance Systems (ADAS) industry, commanding an estimated market share of 65% to 70%. Their System-on-Chips (SoCs) have been integrated into more than 125 million vehicles globally, primarily focusing on Level 1 and 2 autonomous systems . The latest chips from Mobileye, the EyeQ6 series, boast a processing capability of 128 Tera Operations per Second (TOPS), making them well-suited for level 2 autonomous operations. TOPS serves as a metric for quantifying computing operations per second for an AI chip, indicating the number of basic mathematical problems it can solve within a second.
Other global independent chip manufacturers that can provide high-performance autonomous driving chips and have a place in the market include NVIDIA, Renesas, Qualcomm, Huawei, Horizon, and Xinchi Technology.
Over the past few years, prominent chip manufacturers have exhibited a discernible trend towards developing autonomous driving chips that extend beyond Level 3 capabilities. Consequently, the competition for computing power in these chips has intensified significantly. In 2021, NVIDIA unveiled Atlan, a chip specifically designed for Level 5 autonomous driving with a computing power of 1000 TOPS. Just a year later, they introduced Thor, the next-generation chip that boasts even more impressive performance, reaching a remarkable 2000 TOPS. Thor’s computing power is nearly 8 times that of Jetson AGX Orin, its current mass-produced product.
Undoubtedly, one of the key trends in autonomous driving chips is the pursuit of high computing power. As autonomous driving technology advances, vehicles must handle an increasing number of sensors, high-precision map positioning, Vehicle-to-Everything (V2X) information communication, various data fusion, decision-making, and planning algorithm calculations. All these tasks generate large amounts of data, necessitating a computing platform with high capabilities for real-time data processing and analysis.
According to Intel’s calculations, in the era of fully autonomous driving, each car will generate up to 4,000GB of data per day . While Horizon’s data suggests every time the autonomous driving level increases, the required chip computing power will grow dozens of times. L2 autonomous driving only needs 2-2.5TOPS of computing power, while L3 autonomous driving requires 20-30 TOPS. Achieving L4 requires over 200 TOPS, and L5 surpasses 2000 TOPS in computing power requirement.
Computing Power is Not the Only Concern
Level 3 serves as the boundary between fully autonomous driving and assisted autonomous driving, often referred to as ‘conditional autonomous driving’. The industry acknowledges that L3 autonomous driving requires the following capabilities: automatic lane changing, highway navigation-assisted driving, and traffic congestion guidance in urban areas.
Achieving conditional autonomous driving at Level 3 demands the integration of numerous sensors, a robust computing platform, and a software platform equipped with deep learning capabilities. Additionally, it relies on the collaboration of high-precision maps and high-precision positioning.
Without a doubt, the key factor among them is computing power. Perception, decision-making, and execution are the three essential elements of autonomous driving. Insufficient computing power poses a significant challenge for self-driving vehicles, as they rely on processing vast amounts of sensory information to make accurate decisions and execute safe operations.
However, computing power alone is not the only indicator of success; the performance of the software in real-world application scenarios holds greater significance. The belief that the main measure of AI chips is solely based on their absolute computing power is a significant misunderstanding. The focus should be on maximizing computing power efficiency, including factors such as computing power per watt, computing power per dollar, and the efficiency of converting computing power into AI results.
In other words, assessing the effectiveness of self-driving chips cannot solely rely on TOPS value. Instead, it should involve a comprehensive evaluation of their computing capabilities. The autonomous driving system relies on a well-balanced computing system that supports the diverse needs of various sensors. These sensors require a comprehensive range of computing power, including the Central Processing Unit (CPU), Graphics Processing Unit (GPU), Neural Processing Unit (NPU), and Digital Signal Processor (DSP), rather than just peak TOPS power.
In the context of actual mass production, it’s imperative to consider comprehensive factors such as cost and power consumption alongside other considerations. The increase in power can not only reduce the vehicle’s range but also cause the chip to generate more heat, thereby reducing performance. Consequently, achieving the initial theoretical peak computing power becomes unattainable. Therefore, AI chips with low power consumption and high computing power will be the core of chip competition in the high-end autonomous driving stage. Among these, the computing power consumption ratio, also known as energy efficiency, comes forth as an important indicator.
Conclusion
The advent of autonomous vehicles heralds a significant shift in transportation, altering our perceptions and utilization of mobility. As vehicles evolve into interconnected intelligent systems capable of autonomous travel, our streets are poised to become networks of self-driving vehicles, potentially enhancing road safety by minimizing human error. However, this transition is fraught with challenges, requiring substantial advancements in technology, such as processing power, infrastructure, and legislation. As we stand on the cusp of this exciting new era, it’s imperative to grasp the profound implications of this paradigm shift and adapt accordingly. By addressing the technical, infrastructural, and regulatory challenges, we can pave the way for a future where vehicles assume control, reshaping the traditional driver-vehicle dynamics.