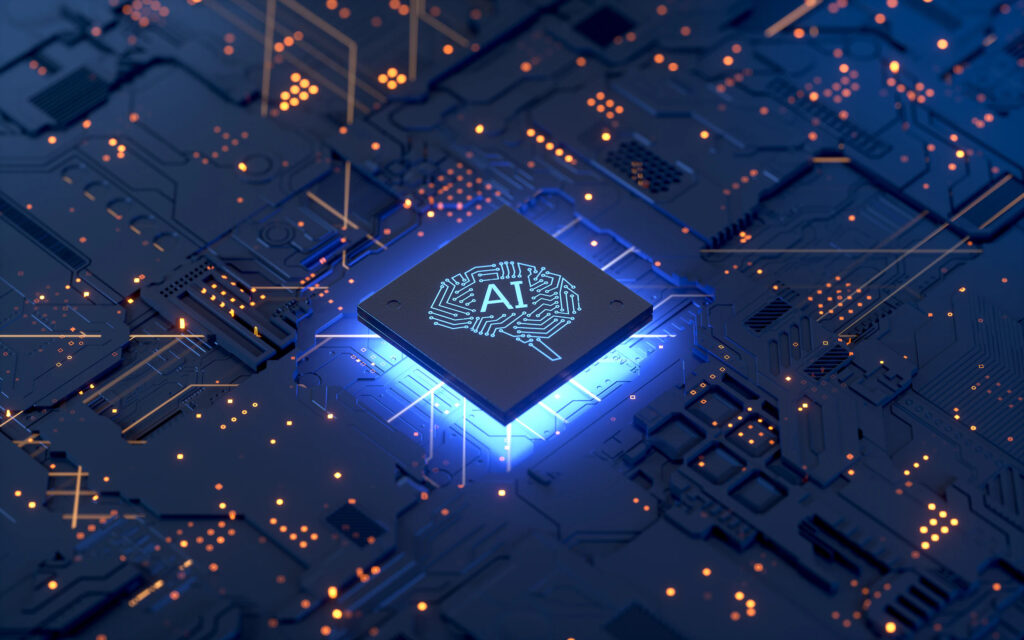
Artificial intelligence (AI) is becoming ever more present in modern-day society. From improving scientific analyses and imaging capabilities to predictive maintenance and monitoring operations in industrial settings, to everyone’s favorite AI chatbot, ChatGPT, AI is starting to take a foothold in many aspects of society; and its capabilities are only limited by the hardware and computing power available to power the software algorithms.
The field of nanotechnology―including both nanomaterials themselves and nanofabrication/nanopatterning methods―is already helping to miniaturize computing components, make electronic components more efficient, and improve the overall power potential for computing technologies (as you can fit more components per area than you can with bulkier materials).
As the computing and technological demands for AI technologies become greater in all aspects of society, the hardware will need to be able to keep up. Nanotechnology is already having an impact in improving the computing sector on all fronts, and this extends to the hardware used for powering and facilitating AI algorithms.
Memristive Devices
Nanomaterials have been gaining a lot of interest recently for memristive devices, and there’s much research coming out using nanotechnology-facilitated memristive devices for many applications. Memristive devices are two-terminal devices―with metal/insulator/metal stack architecture―that act as a resistance switch that can retain a state of internal resistance/conductance based on the history of the applied voltage and current across the device―and most memristors investigated today use nanoscale channels.
Memristive devices have unique physical properties (because of the thin-film and nanoscale materials they are made of) that give rise to fast and low energy switching, conductance monitoring, and a high degree of scalability. There are a number of different memristive devices today that can be used. These include drift memristors, diffusive memristors, and phase change memory (PCM) devices―and are classified into these categories based on the switching materials used in the device and the switching dynamics of the device. It should be noted that the structure of PCM devices is slightly different because there is a phase change layer and insulating layer sandwiched between the metal layers, rather than just an insulating layer, but PCM devices are some of the more mature memristive technologies out there.
Memristive devices tend to have two operation modes―a read mode and a write mode. In the read mode, the conductance is sensed with no disturbance, but during the write mode, the conductance gets programed in the device by applying a voltage that is greater than the device’s threshold―and these read/write voltages get encoded as encoded as pulse trains. PCM devices are slightly different in operation in that they use the phase transitions of the material to change the resistivity across the device―where the material changes from a crystalline material in a low resistivity state to an amorphous material in a high resistivity state.
There are a number of different areas where nanomaterial-based memristors and PCM devices are being used to improve AI hardware. Memristive devices and phase change crossbar arrays have been used for mixed-signal inference engines and in spin-device-based convolution accelerators for convolution neural network (CNN) applications. Memristor devices are also being trialed in a number of deep learning memory devices, including mixed-signal accelerators for deep learning algorithms, as well as in data storage and analog processing operations.
Memristors are also being investigated for storage and processing approaches in processing-in-memory (PIM) architectures to widen the scope of neural network applications. This approach could also have the potential to use a software/hardware interface that would allow developers to produce neural network code to run on the accelerator. Computing-in-memory (CIM) chips using nanoscale memristor devices have also been proposed recently that could help to realize much larger neural network model by reducing the latency and improving the accuracy of the neural networks.
Magnetoelectric Devices
The second big area of nanomaterial-based hardware devices that could have an impact on the accuracy and efficiency of AI algorithms are magnetoelectric devices. Magentoelectric devices are composed of stacked layers of magnetic materials and insulators and work using the alignment of the polarization of the electronic spin in the metal layers. If the polarizations are aligned, the device exhibits a lower resistance, and any higher degrees of resistance are a function of the degrees of misalignment in the polarization. These devices are programmed by either an applied voltage or spin-orbit currents depending on the number of terminals in the device.
Out of all magnetoelectric devices, magnetic tunneling junctions (MTJ) are offering the most promise for AI hardware. While there are a number of devices within the family of MTJs, the majority of them consist of two magnetic layers separated by an insulating layer, with one of the magnetic layers being permanently magnetized to a certain axis and the other layer’s magnetization being altered to provide different resistance values across the device. MTJ device can either be volatile or non-volatile in nature, and their non-volatility makes them a good candidate for use in nanotech-enhanced AI applications. Beyond this, the lower power consumption and sub-nanosecond switching times also make MTJs a promising candidate in AI hardware architectures.
There are a number of areas where MTJs are being investigated for AI applications. One area is in probabilistic models, such as Restricted Boltzmann Machines (RBM) and Deep Belief Networks (DGNs). The architecture of the hardware for these models is layer-by-layer structures for the multiply-accumulate operations of the neural networks used in these models. The implementation of nanoscale materials in these architectures has utilized a crossbar architecture, with both MTJs and memristor systems being employed in the hardware for these models. The architectural design of the hardware shows similarities with CMOS-only architectures, and only differs with the use of MTJ crossbars for multiply accumulate computations.
Another area where MTJs are being investigated and used is Probabilistic Graphical Model (PGM) architectures. The computational cells contain both computational circuits and memory storage―in the form of Conditional Probability Tables (CPTs)―and it is in these CPTs where the non-volatility of MTJs provides a fully connected neural network and low power consumption that prevents latency in the memory access. In some of these architectures, the MTJ devices are taking on the role of both memory storage and computation circuits.
Conclusion
AI continues to get ever more powerful in different aspects of society. Nanomaterials and nanoscale architectures are now being trialed in a range of components―such as memristors, phase change material devices, and magnetic tunneling junctions―that could help to boost a range of neural network and deep learning algorithms. Nanomaterials are already having a big impact on improving and miniaturizing computing hardware, and it could be that AI-specific hardware is one of the next areas of computing that gains significant improvements thanks to nanomaterials.
Source: Mouser Electronics